The Challenges to Overcome to Unlock AI’s Value for Air Cargo
Article submitted by: Rotate – a TIACA Corporate Member
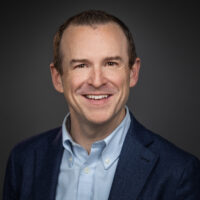
Written by: Ryan Keyrouse, CEO, Rotate
A Brief History of AI in Air Cargo
For a long period, scarcity of data was the air cargo industry’s biggest challenge when it came to advanced data analysis. Recognizing the transformative potential of data-driven insights, substantial investments were directed towards enhancing data and IT infrastructure. As data availability increased, Business Intelligence (BI) dashboards proliferated, sparking enthusiasm about the possibilities of applying Artificial Intelligence (AI) to revolutionize air cargo operations.
Today the industry faces a different challenge: an abundance of data, accompanied by a general frustration regarding the effective utilization of AI to inform decision-making. Management teams are actively seeking practical strategies to harness AI’s capabilities.
The Fundamental Challenge AI Doesn’t Solve
There’s often a misconception that AI in itself will be able to solve some of the industry’s biggest challenges. Over time, it has become evident that AI doesn’t address a foundational issue: airlines must still strategize on how data can optimize commercial decision-making. AI doesn’t replace the need for commercial teams to devise innovative use cases that drive value through better decision-making. AI should be viewed as an enabler rather than an end goal.
The Hidden Wealth of Data at Airlines
A recent survey conducted by Rotate revealed that airlines possess extensive historical data – both internal and market data. However, this data is often underutilized and lacks contextualization for decision-making purposes. Airlines can easily look up their market share or yield position but often then struggle to interpret their competitiveness.
This historical data, deemed ‘boring,’ is frequently overshadowed by / overlooked in favor of the pursuit of real-time metrics like click-to-ship rates, despite lacking immediate practical applications.
Practical Implementation: Fair Share Analysis
Rotate has collaborated with various airlines to determine each airline’s fair share – the expected market share considering their network relative to competitors. Leveraging proprietary capacity data and machine learning algorithms, Rotate incorporates this ‘boring’ data into fair share estimations.
Fair share analysis not only informs airlines about their market position – answering ‘is my market share and yield position good or bad?’ – but also is a required and critical component for optimization of the network and Origin-Destination (OD) sales mix.
Encouragingly, fair share analysis represents just one practical application of AI and data aimed at enhancing commercial decision-making processes.
Ryan Keyrouse is the CEO of Netherlands-based Rotate, the go-to team in the cargo industry for commercial decision-making. His team may or may not have used AI in writing this article.